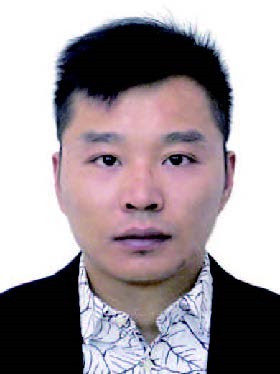
报告时间:2022年6月13日上午9:30-10:30
报告地点:腾讯会议ID:432-575-371
报告人: Hongtian Chen 博士
工作单位:University of Alberta
举办单位:必赢565net官网
报告人简介:Hongtian Chen received the Ph.D. degree in College of Automation Engineering from Nanjing University of Aeronautics and Astronautics, China, in 2019. He had ever been a Visiting Scholar at the Institute for Automatic Control and Complex Systems, University of Duisburg-Essen, Germany, in 2018. Now he is a Post-Doctoral Fellow with the Department of Chemical and Materials Engineering, University of Alberta, Canada. His research interests include process monitoring and fault diagnosis, data mining and analytics, machine learning, and quantum computation; and their applications in high-speed trains, new energy systems, and industrial processes. Dr. Chen was a recipient of the Grand Prize of Innovation Award of Ministry of Industry and Information Technology of the People’s Republic of China in 2019, the Excellent Ph.D. Thesis Award of Jiangsu Province in 2020, and the Excellent Doctoral Dissertation Award from Chinese Association of Automation (CAA) in 2020. He was a guest editor and Youth Editorial Board for several international journals such as IEEE Transactions on Artificial Intelligence and IEEE Transactions on Neural Networks and Learning Systems. He was also Organizing Chairs and Program Chairs of international conferences such as 5th International Conference on Robotics, Control and Automation Engineering.
报告简介: The increased complexity and intelligence of automation systems require the development of intelligent fault diagnosis (IFD) methodologies. By relying on the concept of a suspected space, this study develops explainable data-driven IFD approaches for nonlinear dynamic systems. More in detail, we parameterize nonlinear systems through a generalized kernel representation used for system modeling and the associated fault diagnosis. An important result obtained is a unified form of kernel representations, applicable to both unsupervised and supervised learning. More importantly, through a rigorous theoretical analysis we discover the existence of a bridge (i.e., a bijective mapping) between some supervised and unsupervised learning-based entities. Notably, the designed IFD approaches achieve the same performance by the use of this bridge. In order to have a better understanding of the results obtained, unsupervised and supervised neural networks are chosen as the learning tools to identify generalized kernel representations and design the IFD schemes; an invertible neural network is then employed to build the bridge between them. This study is a perspective article, whose contribution lies in proposing and detailing the fundamental concepts for explainable intelligent learning methods, contributing to system modeling and data-driven IFD designs for nonlinear dynamic systems.
文 许水清 审核 赵吉文